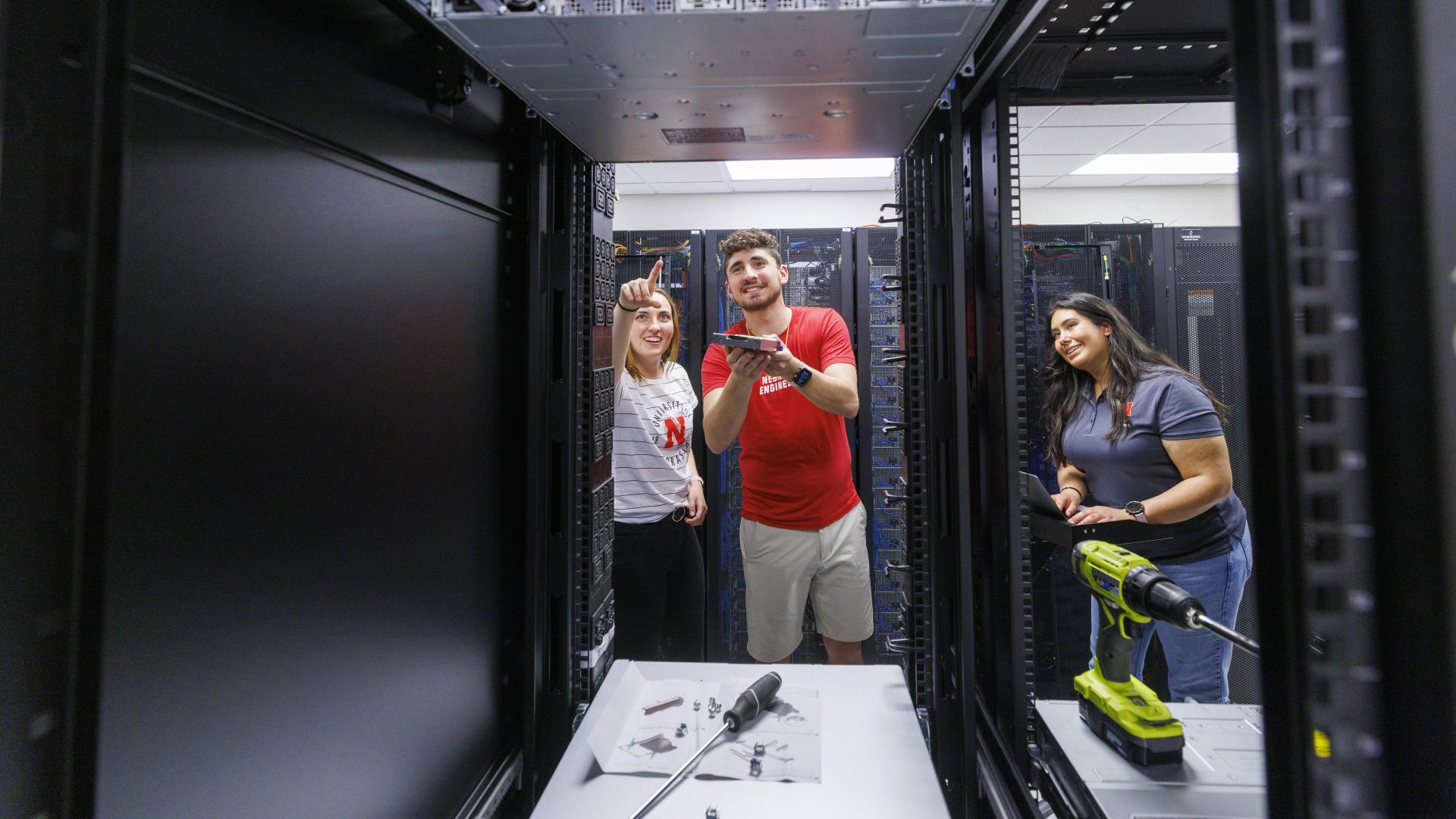
The requirements for our M.S. in Computer Science (Computer Engineering Specialization) have been updated for new students and current students who choose our new option. Review the new requirements here.
READY TO APPLY?
Recent News
Instructions for resetting your password
If you are unable to login after the upgrade or wish to change your password before the upgrade please do the following:
Log in to the School of Computing Account Management Utility (AMU) (https://cse.unl.edu/account) with your account credentials and then clicking on the change password link from the navigation links on the left.